Customer feedback allows you to understand your audience, identify areas for improvement, and ultimately, build stronger relationships. But in today’s data-driven world, navigating the vast amount of customer feedback can be overwhelming.
This is where the AI feedback loop comes in, offering a transformative approach to unlocking deeper customer insights and driving meaningful business decisions.
Imagine a system that not only analyzes customer feedback but also learns from it. That's the magic of AI feedback loops. By continuously processing and continual learning from sentiment analysis, text analysis, opinion mining, and even emotion detection, these loops transform customer feedback into actionable insights.
In this blog, we will talk about AI feedback loops, their working technique, how they help enhance feedback analysis, their practical applications, and how you can optimize these loops to leverage business benefits.
TL;DR
- The AI Feedback Loop offers a transformative approach to understanding and responding to customer feedback in real time.
- By leveraging AI-powered techniques like sentiment analysis, text analysis, and emotion detection, businesses can extract actionable insights from feedback data, driving continuous improvement and enhancing customer satisfaction.
- Implementing AI feedback loops requires a strategic approach, including data quality assurance, ethical considerations, and continuous optimization.
- With the right tools and methodologies, businesses can unlock the full potential of AI in customer feedback management, gaining a competitive edge and fostering long-term success.
- Zonka Feedback offers a complete suite of AI capabilities to help create and close the feedback loop. Its AI capabilities can allow businesses to fetch actionable insights, customer sentiments, and emotions from new data. It learns from the feedback and improves the offerings to enhance the customer experience. You can sign up for a free 14-day trial.
Measure Customer Feedback & User Insights
With powerful surveys, understand what your customers feel & need and learn ways to delight them.
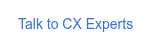
What is the AI Feedback Loop?
An AI feedback loop is a powerful tool that allows AI systems to continuously learn and improve over time. It is a cyclical process that allows any AI system to continuously learn and improve over time. It works like a closed loop system, where the AI model interacts with its environment and learns from the results.
It works like a cycle, where the AI system:
- 1. Receives data: This data can come from various sources, including user interactions, sensor readings, external databases, or even other AI systems.
- 2. Analyzes the data: The AI uses machine learning algorithms to analyze the data, identify patterns, and extract insights. This could involve tasks like sentiment analysis, anomaly detection, or predicting future outcomes.
- 3. Generates output: Based on the analysis, the AI generates an output. This could be a recommendation, a decision, a creative text format, or simply updated knowledge within the system.
- 4. Receives feedback: The AI then receives feedback on its output. This feedback can come from human users, other AI systems, or by comparing the output to real-world outcomes.
- 5. Learns and improves: Finally, the AI learns from the feedback and uses it to improve its future performance. This could involve adjusting its algorithms, updating its knowledge base, or refining its decision-making process.
Understanding AI Feedback Loops in Customer Feedback Management
AI feedback loops fundamentally involve a recurring cycle in which an AI model’s outputs are persistently gathered, scrutinized, and employed for its own enhancement or retraining, thereby facilitating continuous learning and performance progress. In the context of customer feedback management, these loops are invaluable.
An AI feedback loop in the context of customer feedback analysis refers to a process where an Artificial Intelligence (AI) system learns and improves its ability to understand and analyze customer feedback over time.
They leverage data from user interactions to train AI models, ensuring that products, services, or businesses evolve in line with customer needs and preferences.
These feedback loops work by gathering system outputs, which could come from a variety of sources, including feedback received through surveys, human inputs, and even other AI systems. This collection of feedback data enables the AI model to steer subsequent learning and system refinement, creating a closed loop learning system that is continually improving.
Adopting AI feedback loops paves the way for decisions backed by more data and a user experience that is more intuitive. By actively correcting model biases, promptly addressing system issues, and integrating valuable human feedback, businesses can ensure their machine learning models and services stay in line with customer expectations.
AI Feedback Loops for Customer Feedback Management
AI feedback loops in customer feedback management software can go beyond simply analyzing data and can learn and improve over time. This is achieved through a specific type of AI learning called "closed-loop learning."
Here's a breakdown of how it works in this context:
Step #1. Sentiment and Emotion Detection
The AI system begins by analyzing customer feedback for sentiment and emotion. This involves techniques like Natural Language Processing (NLP) to understand the meaning and emotions behind the customer's words.
Step #2. Actionable Insights and Recommendations
Based on the sentiment analysis, the AI generates actionable insights and recommendations. This could involve:
- Identifying common themes and trends: The AI system can identify recurring issues, suggestions, and areas of improvement mentioned by customers.
- Prioritizing issues: The AI can prioritize issues based on their severity, frequency, and potential impact on customer satisfaction.
- Suggesting solutions: The AI can recommend potential solutions to address the identified issues, drawing from internal data and best practices.
Step #3. Action Taken and Feedback on Results
The business then takes action based on the AI's recommendations. This could involve implementing changes to products, services, or customer support processes.
Step #4. Feedback Loop and Improvement
Here's where the "closed-loop" aspect comes in:
-
Monitoring customer responses: The business monitors customer response and feedback to the implemented changes. This can be done through new feedback, surveys, or tracking key performance indicators (KPIs) like customer satisfaction scores
-
Evaluating the impact: The AI analyzes the feedback on the implemented actions to understand if they effectively addressed the identified issues. This could involve:
-
Analyzing new customer feedback to see if the sentiment has improved.
-
Tracking changes in KPIs related to the addressed issues.
-
-
Continuous Learning: Based on the evaluation, the AI system "learns" from the results. This allows it to refine its future analysis and recommendations in the following ways
-
Improving sentiment analysis accuracy: By understanding how customer sentiment changed after taking action, the AI can improve its ability to interpret future feedback accurately.
-
Refining recommendations: Analyzing which recommendations led to positive outcomes helps the AI refine its future suggestions for better effectiveness.
- More accurate insights: The AI becomes better at identifying customer needs and concerns.
- More effective recommendations: The AI suggests solutions that are more likely to address customer issues and improve satisfaction.
- Overall improvement in customer experience: By effectively addressing customer feedback and implementing appropriate changes, businesses can drive higher customer satisfaction and loyalty.
-
By effectively implementing and monitoring AI feedback loops with a responsible and human-centric approach, businesses can leverage them to gain deeper customer insights, improve their customer experience, and achieve continuous improvement in their products and services.
Benefits of Implementing AI Feedback Loops
A principal advantage of AI feedback loops is the improvement in model precision. Much like how students learn from graded assignments, AI models learn from their errors, continually refining their performance. Moreover, these feedback loops allow AI systems to adjust to changing environments, such as fluctuating market demands, contributing to sustained performance improvements.
- Improved customer satisfaction: By addressing customer feedback promptly and effectively, businesses can enhance the overall customer experience and build loyalty.
- Enhanced product/service quality: By leveraging insights gleaned from feedback data, businesses can identify areas for improvement and innovate their offerings to better meet customer expectations.
- Increased operational efficiency: The automation capabilities of AI streamline the feedback analysis process, allowing businesses to glean insights faster and take action more efficiently.
- Competitive advantage: Organizations that embrace the AI feedback loop gain a strategic edge by staying attuned to market trends, customer preferences, and emerging opportunities.
- Improved user experience: Experience is substantially enhanced by AI feedback loops. For instance, search engines leveraging AI feedback loops can offer more pertinent results, leading to a positive cycle of user engagement and data refinement. Similarly, conversational AI that employs feedback loops can deliver more bespoke support by assimilating industry-specific data and past user interactions.
- Feedback-driven personalization: Businesses can deploy strategies that enable more captivating and tailored experiences for customers. With the application of feedback, AI systems develop the capacity to evolve and better serve user needs, detecting new support topics, and guiding message rerouting. This instigates a virtuous cycle of continuous improvement within AI models, wherein enhanced services based on customer data feedback result in higher satisfaction and further data collection.
Challenges in AI Feedback Loop Deployment
Despite the substantial benefits of AI feedback loops, their implementation comes with its own set of hurdles.
A key issue lies in the potential to inadvertently perpetuate existing biases if the algorithms are trained on biased datasets. This can lead to the reinforcement and amplification of those biases, skewing the AI’s understanding of user needs and preferences.
Moreover, simply adding more algorithms to the AI models cannot automatically detect and correct biases. Overlooking the ethical dimensions of technology may lead to technological solutionism, where technology is seen as the sole solution to complex societal issues.
The intricacy of AI feedback loops concurrently presents obstacles in terms of explainability. AI systems must provide decisions that are not only correct but also intelligible to earn user trust. This is vital as a lack of clarity on how AI systems arrive at decisions can result in ineffective feedback loops.
After all, user feedback may be compromised if the AI’s decision process is not clear. As such, the explanations provided by AI systems must be comprehensible to a broad range of stakeholders and not just AI specialists.
Finally, guaranteeing the quality of data employed in AI feedback loops is paramount. Training AI with diverse, high-quality data is vital for avoiding biases and fostering the robustness of AI models within feedback loops. Moreover, ensuring the reliability of AI model outcomes and mitigating potential problems in production data is crucial to maintaining the integrity of AI feedback loops.
AI Functionalities in Survey Software
Survey software has witnessed an evolution in AI functionalities, now encompassing a broad selection of tools and features designed to capture and comprehend user feedback in progressively intricate ways.
For instance, sentiment analysis tools utilize machine learning and natural language processing (NLP) to discern the emotional undertones in textual feedback.
Similarly, text analysis helps capture and categorize information from text data, facilitated by NLP’s ability to parse human language and extract valuable insights.
Large language models, as a part of NLP, play a significant role in this process.
Emotion detection or analysis, an advanced feature of some sentiment analysis tools, provides a deeper understanding of specific emotions customers express in their feedback, enhancing basic sentiment analysis. Moreover, cutting-edge sentiment analysis capabilities enable the recognition of:
- Context
- Slang
- Specialized industry terms
- Nuanced language such as sarcasm
This is paramount for accurately interpreting customer sentiments.
AI functionalities also extend to tools like opinion mining, intent analysis, and emotion detection, which collectively help in discerning not just surface sentiment but also deeper customer intentions and feelings.
Techniques like topic detection, categorization, and topic modeling organize feedback into related themes, enabling structured insights. Metrics like recall and precision guide the measurement of text analysis accuracy.
With the rise of AI-generated content, these techniques become even more crucial in understanding and analyzing the vast amount of ai generated data available, making the use of an AI system essential.
Furthermore, these tools must offer:
- Seamless integration with existing business systems
- Efficient, actionable data insights derived from text data collected from varied sources
- Monitoring of a broad spectrum of online sources, encompassing social media, blogs, and other channels
This ensures that a company’s feedback analysis is wide-ranging and comprehensive.
Optimizing Your AI Feedback Loop
The optimization of AI feedback loops requires a blend of strategies with the aim to guarantee both the quality and diversity of feedback data. One approach is to collect diverse feedback by implementing brief and frequent feedback cycles that engage a broad range of stakeholders. Balancing the use of quantitative and qualitative questions and catering to various communication preferences allows for a richer and more diverse set of feedback data. Moreover, effective survey questions are characterized by:
- Clarity and relevance to the AI project’s objectives
- Asking participants for concise, impartial feedback
- Sent through multiple channels, including anonymous options.
Strict attention to data quality and security is also crucial. This is achieved through:
- Actionable responses to feedback
- Embedding feedback mechanisms in all customer interactions
- Surveys that are mobile-friendly to support wider accessibility and improve completion rates
These measures further contribute to the security and integrity of feedback data.
Adopting a responsive strategy to customer feedback ensures that businesses align their actions with customer expectations, trust, and engagement. Segmenting feedback and focusing on priority areas helps in efficient action planning and transparent communication of results and actions, fostering continuous improvement.
Furthermore, collaboration between AI practitioners and domain experts is essential to ensure feedback is properly utilized for model refinement and strategy enhancements.
Visualizing AI Feedback Loops
Visualization of AI feedback loops can yield an insightful understanding of your AI system’s effectiveness and the prevailing trends in customer feedback. Visual elements such as charts and graphs in sentiment analysis help track changes in customer opinion over time, providing a clear, intuitive view of data trends.
Such visual aids can effectively illustrate the segmentation of generated data into training, validation, and testing sets, providing a clear representation of the process that underpins the maintenance of the accuracy and reliability of AI models, while preventing model collapse. By adjusting model parameters, the model performance of these AI models can be further optimized.
Integrating AI Feedback Loops into Your Business Strategy
Integrating AI feedback loops into your business strategy can significantly uplift different facets of your operations. For instance, AI marketing tools like Salesforce’s Einstein GPT and HubSpot’s CRM utilize vast data points to provide accurate predictions, personalized communications, and efficient interactions without manual data entry.
AI also enables businesses to provide personalized experiences. Amazon’s product recommendations and eBay’s customized browsing experience through 3D product renderings exemplify how businesses can leverage feedback loop data to tailor their services to individual user preferences.
Moreover, AI feedback loops can also enhance customer service and optimize revenue.
For example, Airbnb uses AI for background checks via social media scanning and employs machine learning for intelligent pricing strategies, demonstrating the application of AI in user vetting and revenue optimization. Similarly, IBM Watson Assistant and SEMrush’s AI tools exemplify the use of AI in providing more accurate and efficient customer service and real-time feedback on content for marketers.
Start Integrating AI Feedback Loop
In conclusion, AI feedback loops are transforming the way businesses understand and respond to customer feedback. By leveraging these technological advancements, businesses can enhance their decision-making processes, improve user experiences, and adapt to changing market demands. While challenges such as data bias and explainability remain, the potential benefits and future advancements of AI in customer feedback management make it an exciting area for businesses to explore and invest in.
Zonka Feedback is a powerful survey tool that allows businesses to integrate their customer feedback management with AI capabilities.
This AI survey tool offers several features and functionalities for businesses to derive the best data from customer feedback. It includes a sentiment analysis tool and text analysis tool with analysis based on advanced AI techniques like opinion mining, text mining, intent analysis, emotion detection, and much more.
You can sign up for a free 14-day trial or simply schedule a demo to understand how the product’s AI capabilities or its feedback loop works.
Frequently Asked Questions
1. What is AI feedback?
AI feedback refers to the use of AI tools to automate and analyze customer feedback, helping companies understand customer behavior patterns, needs, and preferences. Additionally, AI feedback can also involve the use of feedback loops, where AI models improve accuracy over time by learning from errors.
2. What are the 4 elements of any feedback loop?
The four elements of any feedback loop are stimulus, sensor, control center, and effector. These components are crucial for the operation of a negative feedback loop.
3. What is a learning feedback loop?
A learning feedback loop is a two-way conversation between students and teachers that encourages student agency and allows students to actively participate in their learning process. It helps them learn how to learn.
4. What is a feedback loop example?
A feedback loop example is when a retail company collects customer feedback on pricing, adjusts prices, monitors the market, and then gathers feedback to ensure customer satisfaction and sales have improved. This type of loop allows for continuous improvement in response to customer input.
5. What are the benefits of AI feedback loops?
AI feedback loops offer numerous benefits, including enhancing model accuracy, adapting to changing environments, and delivering personalized user experiences. These advantages contribute to the overall improvement of AI systems. By continuously analyzing and integrating user feedback, AI models can refine their algorithms to better meet user needs, ensuring a more seamless and intuitive interaction.
Furthermore, as conditions and user behaviors evolve, AI feedback loops empower these systems to adjust dynamically, maintaining relevance and efficacy. This leads to a more engaging and satisfying experience for users, as they receive services that are tailored to their preferences and requirements. Ultimately, the iterative process of feedback loop-driven learning is integral to the development of sophisticated, user-centric AI applications.