Introduction
For years, organizations have been gathering vast amounts of unstructured data from surveys, online reviews, Net Promoter Score (NPS) responses, support tickets, chat logs, market research, employee feedback, product reviews, and much more.
However, they often struggle to analyze all this information effectively, leaving valuable customer insights buried and unused.
This is where thematic analysis comes in. It transforms scattered, text-heavy data into meaningful themes and subthemes, enabling businesses to identify trends, address concerns, and make informed decisions. Instead of manually sifting through countless documents and platforms, organizations can now uncover patterns efficiently, saving time and resources.
Not just that—with advancements in artificial intelligence (AI) and Natural Language Processing (NLP), this process has become even more streamlined. Automated thematic analysis delivers insights in minutes, empowering businesses to act quickly, anticipate changes, and make data-driven decisions.
But how does it work, and why is it being widely adopted by leading businesses, researchers, and analysts?
Let’s dive in.
Eliminate Guesswork with AI-Driven Thematic Analysis
Spot patterns, understand sentiment, and make informed business decisions. Thematic analysis links customer insights to measurable outcomes like CSAT, NPS, and churn rate.
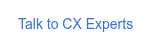
What is Thematic Analysis?
Thematic analysis is a qualitative research method used to identify, analyze, and report recurring patterns—known as themes—in unstructured textual data. It helps businesses extract meaningful insights from large volumes of customer feedback, support conversations, product reviews, and other qualitative inputs.
Thematic Analysis Definition: It refers to the process of coding qualitative inputs to uncover hidden meanings and structure the data into well-defined themes.
At its core, thematic analysis process follows a structured yet flexible process that transforms unstructured text into meaningful categories. Here’s how it works:
- Data Collection: Gathering qualitative inputs from surveys, chatbot logs, support tickets, and online discussions.
- Pattern Recognition: Identifying recurring ideas, underlying sentiments, and emerging concerns.
- Theme Development: Grouping related patterns into broader themes that highlight key trends and opportunities.
- Actionable Insights: Using these themes to drive decisions in customer experience, product innovation, and business strategy.
Qualitative vs. Quantitative Data in Thematic Analysis
Thematic analysis is designed for qualitative data—open-ended survey question responses, interviews, and unstructured feedback—where context and interpretation matter. While quantitative data reveals what is happening through numbers and trends, thematic analysis uncovers the why by interpreting words, emotions, and experiences that numbers alone can’t explain.
Why Thematic Analysis Matters?
Numbers alone cannot explain the reason behind customer sentiment. A drop in NPS or CSAT scores tells a company that satisfaction is declining, but it does not reveal why. Thematic analysis fills this gap by:
- Identifying Core Issues and Emerging Needs: AI customer feedback analysis pinpoints persistent complaints, commonly praised features, and new requests, enabling businesses to enhance products and services effectively.
- Adding Depth to Performance Metrics: Scores indicate trends, but thematic analysis uncovers the real drivers behind customer sentiment, whether frustration with response times, appreciation for usability, or expectations for future improvements.
- Anticipating and Preventing Setbacks: Instead of reacting to problems, businesses detect early warning signs, refine operations, and elevate experiences before issues impact retention.
Businesses that implement AI-powered thematic analysis don’t just gain better insights—they gain a competitive and financial edge. According to KPMG's Global Customer Experience Excellence Report, for every $1 invested in AI, businesses see an average return of $3.5, with 5% of companies reporting a return of $8, proving that leveraging AI-driven insights leads to smarter, more profitable decision-making.
Why is Thematic Analysis Critical for Businesses?
Advancements in AI and NLP help businesses analyze vast amounts of unstructured data quickly and accurately to optimize services and enhance customer experience management.
Here are the key benefits:
- Detecting Recurring Issues & Sentiment Shifts: Businesses monitor experience data to track emerging concerns, sentiment trends, and areas for improvement.
- Reducing Manual Workload: Automating data categorization frees teams to focus on deeper analysis instead of manually sorting large datasets.
- Enhancing Customer Experience: By identifying service gaps, product pain points, and inefficiencies, businesses implement targeted solutions to improve customer satisfaction.
By leveraging AI-driven thematic analysis software, organizations stay ahead of trends, refine strategies, and improve customer satisfaction with precision.
Where does Thematic Analysis Apply?
Thematic analysis is not limited to one department—it is a versatile qualitative data analysis software used across various business functions to unearth actionable insights. Here are some key applications:
- Customer Experience (CX): Thematic analysis in customer experience pinpoints service gaps and pain points affecting satisfaction.
- Product Development: It uncovers feature requests and usability concerns to refine the roadmap.
- Support Operations: It identifies recurring issues to enhance efficiency and resolution times.
- Marketing Strategy: It aligns messaging with customer expectations and sentiment to improve campaign effectiveness.
- Social Research: Reflexive Thematic Analysis, a qualitative method based on researcher interpretation, is used to explore human experiences and cultural patterns in interviews, focus groups, and ethnographic data.
What Are Themes in Thematic Analysis?
A theme in thematic analysis is a recurring pattern of meaning that emerges from qualitative data. It represents core ideas, trends, concerns, perspectives, opportunities, or experiences shared across a dataset, helping researchers and businesses uncover what truly matters. Themes are developed through coding, where specific segments of text are labeled to capture key insights.
For example, in product reviews, themes might include:
- Ease of use: Customers frequently mention a simple interface or intuitive navigation.
- Value for money: Discussions around pricing, affordability, and product quality.
For themes to be meaningful, they must align with the entire dataset and accurately represent the most significant findings. By recognizing and structuring these themes, businesses and researchers can draw precise conclusions, anticipate needs, and make meaningful decisions that drive real impact.
One-Level vs. Two-Level Thematic Analysis
Thematic analysis can be conducted at varying levels depending on how much depth is required. One-level analysis categorizes data into broad themes, offering a high-level overview, while two-level analysis introduces subthemes for deeper insights, making it easier to pinpoint specific customer concerns and take targeted action.
One-Level Thematic Analysis (Single-Layer Broad Themes Only)
A one-level approach groups qualitative data into general themes without further breakdown. It’s useful for spotting high-level trends but lacks the specificity needed to fully understand underlying issues.
For example, a company analyzing customer reviews finds the following recurring complaints:
- “I waited 30 minutes to speak to someone.”
- “My issue wasn’t resolved after multiple calls.”
- “The agent gave a generic response.”
- “I was billed twice for the same subscription.”
- “Still no refund and the invoice total doesn’t match my purchase.”
These reviews could be categorized under broad themes like:
- Customer Support Issues
- Billing Complaints
While these themes highlight problem areas, they don’t explain whether dissatisfaction stems from long wait times, ineffective resolutions, or unhelpful responses. Teams would need to manually dig deeper to address specific concerns.
Two-Level Thematic Analysis (Themes & Subthemes)
A two-level approach refines broad themes into subthemes, adding clarity and detail. This structure makes it easier to identify precise customer pain points and respond strategically.
Refining the previous themes into subthemes might look like this:
- Theme: Customer Support Issues
- Subtheme: Long wait times – Delays in response or long hold times.
- Subtheme: Unresolved issues – Issues remain open with no resolution.
- Subtheme: Unhelpful responses – Agents provide scripted or vague solutions.
- Theme: Billing Complaints
- Subtheme: Unexpected charges – Fees customers weren’t informed about.
- Subtheme: Refund delays – Slow processing of refund requests.
- Subtheme: Invoice errors – Billing discrepancies causing confusion
This structure enables businesses to act on specific issues rather than making general assumptions. Instead of just knowing that customers are unhappy with billing, companies can see that refund delays are a recurring issue—and implement faster processing.
Similarly, if long wait times in customer support emerge as a major subtheme, leadership can focus on hiring more agents, improving automation, or optimizing response workflows.
How do Themes and Subthemes Work Together?
Two-level thematic analysis structures data into two layers:
- Themes (Main Categories): These represent broad topics that reflect customer concerns, feedback patterns, or pain points.
- Subthemes (Subcategories): These subthemes also known as subtopics, provide a deeper level of insight by identifying the specific underlying issues within each theme.
This layered approach ensures businesses can distinguish between general concerns and specific issues, helping teams focus on what matters most.
Why Two-Level Analysis Is More Effective?
Two-level thematic analysis improves decision-making by:
- Pinpointing specific customer pain points: Enabling teams to prioritize and take targeted action.
- Tracking trends over time: Monitoring how concerns evolve to address issues before they escalate.
- Improving customer retention: Addressing root causes rather than surface-level frustrations to build loyalty.
This enables faster issue resolution, better product enhancements, and more effective customer support strategies, ultimately improving customer satisfaction.
Themes vs. Topics: Different Terminology in Thematic Analysis
Themes and topics are sometimes used interchangeably in qualitative analysis, with manual methods favoring themes and AI-based tools using topics. Despite the terminology, both approaches serve the same purpose—structuring unstructured data for deeper insights.
Traditional thematic analysis organizes data into themes and subthemes, while AI-based tools label them as topics and subtopics. Though the wording differs, both methods categorize qualitative information in a way that makes patterns, concerns, and trends more visible.
For instance, a billing-related complaint may be labeled as:
- Manual Analysis: Theme: Billing Issues
- Subtheme: Incorrect Amount
- AI-Based Analysis: Topic: Billing Problems
- Subtopic: Pricing Discrepancy
Regardless of terminology, both approaches help businesses uncover key trends, identify customer concerns, and take data-driven action to improve products, services, and overall experience.
When to Use Thematic Analysis?
Thematic analysis is widely used across various industries, enabling organizations to identify pain points, track trends, and make data-driven decisions. Here’s how different sectors apply it to extract valuable insights:
The Six-Step Process of Thematic Analysis
Braun and Clarke’s six-step framework provides a systematic way to break down large volumes of text, making it easier to recognize trends and optimize services.
Step 1: Familiarization with Data
The first step is to review qualitative data—customer surveys, call transcripts, and reviews—to grasp overall experiences and sentiment. This involves actively reading or listening multiple times to identify repeated phrases, emotions, and observations.
Here are the key questions to consider:
- Are there recurring words, complaints, or compliments?
- Do customers express emotions like frustration, excitement, or confusion?
For example, if multiple reviews mention “long wait times,” “no response from support,” or “waiting days for a reply”, this signals a pattern of service delays that may require further analysis.
Step 2: Generating Initial Codes
Once familiar with the data, the next step is assigning codes—short labels summarizing key insights without changing their meaning. This process helps categorize feedback into structured elements, making it easier to identify patterns later.
To generate codes, break down data into smaller units and tag them based on the issue described. These codes will later be grouped into broader themes.
For example:
1. Long wait times:
- Customer complaint:
- “I had to wait 30 minutes on hold before speaking to support.”
- Code Assigned:
- Long wait time
2. Faulty product and replacement issues:
- Customer complaint:
- “The product stopped working after a week, and I couldn’t get a replacement.”
- Codes Assigned:
- Product defect (indicating a quality issue)
- Replacement difficulty (highlighting service inefficiency)
At this stage, focus on capturing key details efficiently without overanalyzing—these initial codes will later form the foundation for broader themes.
Step 3: Searching for Themes
Once coded, related issues are grouped into themes—broader categories representing key feedback patterns. This step helps businesses structure qualitative data in a way that highlights major trends and recurring concerns.
For example, if multiple codes relate to customer service delays, such as:
- Long wait time
- No update on the ticket
They can be grouped under the theme: "Support Delays"
Similarly, if codes related to billing issues include:
- Refund taking too long
- Unexpected extra charges
They can be categorized under “Billing Concerns”
At this stage, look for relationships between different codes and ensure each theme accurately reflects a meaningful trend in customer responses.
Step 4: Reviewing Themes
Once themes are created, they are refined by checking for overlap, ensuring clarity, and verifying alignment with the original data. This ensures that themes accurately represent key trends and are meaningful for decision-making.
- Merging Similar Themes:
If two themes are closely related, they should be combined for clarity.
-
- For example: "Delayed Response" and "No Updates on the Ticket" can be merged into Support Delays to avoid redundancy.
- Breaking Down Broad Themes:
If a theme is too general, it can be split into subthemes for more precise insights.
-
- For example: Instead of a broad theme like Customer Experience Issues, refine it into:
- Long Support Wait Times
- Unhelpful Agent Responses
- Unresolved Issues
- For example: Instead of a broad theme like Customer Experience Issues, refine it into:
By ensuring themes are well-structured and clearly defined, businesses can extract deeper insights and make informed improvements.
Step 5: Defining and Naming Themes
Once themes are refined, they need clear and specific names to ensure consistency across teams. Well-defined themes make it easier to interpret findings and take action.
Here’s how to name themes effectively:
- Be precise: Avoid vague or overly broad labels.
- Use actionable terms: Ensure the name directly reflects the issue.
- Keep it simple: Use clear language that everyone can understand.
A few examples of effective theme naming:
- Instead of "Service Issue", use "Long Wait Times in Support"
- Instead of "Billing Problem", use "Refund Delays"
Not just that. Each theme should also include a brief definition for clarity. For instance, if there is the theme of “Refund Delays”, the following would be its definition:
- Definition: Customers experience extended wait times for refunds after cancellations or incorrect charges.
By using concise, well-structured names and definitions, businesses can ensure themes are easily understood and drive targeted improvements.
Step 6: Presenting Insights and Taking Action
The final step is to present findings in a way that enables meaningful improvements. Thematic analysis is not just about organizing data; it should drive strategic actions that enhance business operations and customer experience.
Insights can be shared through dashboards, reports, or visual charts that highlight key trends. Clearly communicating these findings ensures that decision-makers can act on them effectively.
For example, if support delays are a recurring theme, the company may hire more agents or implement automation to improve response times. If unclear pricing policies emerge as a major issue, the marketing team could refine communication around costs to reduce confusion.
By transforming qualitative data into actionable insights, businesses can address core issues, improve services, and enhance customer satisfaction in a data-driven way.
Types of Thematic Analysis
Different approaches to thematic analysis influence how data is categorized, structured, and interpreted, depending on research objectives and data complexity. The choice of method impacts how themes are identified, analyzed, and applied to generate meaningful insights.
1. Inductive vs. Deductive Approaches: How Are Themes Identified?
Thematic analysis can be inductive, where themes emerge naturally from the data, or deductive, where analysis follows a predefined framework. The choice depends on whether the goal is to discover new insights or validate existing ones.
Inductive Approach: Themes Develop Organically
Themes arise directly from the data without predefined categories, making this approach ideal for exploratory research. This approach is ideal for doing exploratory research.
💡 For example, you receive multiple customer reviews after a product launch:
- "The checkout process is confusing."
- "I couldn’t find the promo code box."
- "The total cost wasn’t visible until the last step."
Without predefined categories, themes develop organically, helping uncover unexpected customer pain points. Further analysis might reveal subthemes such as unclear pricing, promo code issues, and checkout navigation difficulties.
Deductive Approach: Using a Predefined Framework
This method categorizes data based on existing theories, business goals, or industry benchmarks, ensuring structured analysis. It is best for structured reporting and hypothesis validation.
💡 For example, your company already has a framework for analyzing checkout-related feedback:
- Predefined Theme: Friction in the Checkout Process
- Subthemes: Usability Issues, Payment Process
Each new response is categorized into existing themes instead of forming new ones, ensuring consistency in tracking trends over time. This structure aligns analysis with business objectives and enables comparison with past research.
2. Semantic vs. Latent Approaches: How Deep Should the Analysis Go?
Once themes are identified, they can be analyzed at different levels—either focusing on explicit content or uncovering underlying meanings.
Semantic Approach: Direct Meaning
Semantic analysis examines explicit statements without interpretation. Researchers categorize data based on what is directly said, making this method useful for summarizing common concerns in structured feedback.
💡 For example, if multiple customers mention "slow response time," that phrase itself becomes a theme. This straightforward approach is efficient for tracking trends in survey responses, reviews, or support logs.
Latent Approach: Deeper Interpretation
This approach uncovers hidden motivations behind what people say, revealing deeper customer behaviors and expectations. It moves beyond direct phrases to explore underlying emotions, attitudes, and assumptions.
💡 For example, if customers frequently mention "fast delivery," latent analysis may reveal that speed is valued due to past negative experiences or cultural preferences. This deeper insight helps businesses understand not just what customers say but why they feel a certain way.
3. Hybrid Approach: The Best of Both
For flexibility, a hybrid approach combines inductive and deductive methods, offering a balance between structure and discovery.
Researchers may start with a predefined framework (deductive) but remain open to identifying new themes (inductive) that emerge during analysis. This is particularly useful when organizations need structured reporting but also want to capture unexpected insights that could inform strategy.
4. Reflexive vs. Codebook Approaches: Managing the Coding Process
Another key distinction in thematic analysis lies in how themes are coded and refined. The two primary approaches are reflexive and codebook-driven, each offering different levels of flexibility.
Reflexive Approach: Evolving Themes and Codes
This iterative process allows themes and codes to evolve, making it ideal for complex qualitative data requiring deeper interpretation. Researchers continuously refine themes based on new insights, allowing for greater adaptability.
💡 For example, a tech company analyzing user feedback may initially categorize complaints about app performance under "Usability Issues." As analysis progresses, researchers refine themes further, identifying subthemes like "App Crashes," "Slow Load Times," and "Navigation Difficulties."
Codebook Approach: Structured and Consistent Coding
This structured approach is best for large-scale studies or team-based research, ensuring consistency, replication, and easier comparison. Analysts follow a predefined set of themes and codes, reducing subjectivity in the interpretation process.
💡 For example, a bank analyzing customer complaints may use a predefined framework with categories such as "Account Issues," "Transaction Errors," and "Loan Processing Delays." Since the coding structure remains consistent, comparisons across different datasets become more reliable.
Choosing between these approaches depends on the need for flexibility versus the importance of maintaining standardized categorization for long-term analysis.
A Quick Comparison of the Different Approaches
Here’s a simple breakdown to help you choose the right approach based on your needs.
Approach | What It Means | Best For | Example |
Inductive vs. Deductive | Themes emerge naturally vs. Predefined themes guide analysis | Exploring unknown issues vs. Validating known concerns | A retailer uncovers confusing return policies (inductive), while a telecom company categorizes comments into billing errors and network issues (deductive). |
Semantic vs. Latent | Identifying what’s explicitly said vs. Interpreting deeper meanings | Quick insights vs. Understanding customer emotions | Customers say "slow service" (semantic), but deeper analysis shows they feel "ignored and unvalued" (latent). |
Reflexive vs. Codebook | Adjusting themes dynamically vs. Following a fixed framework | Capturing evolving trends vs. Ensuring consistency | A tech company refines themes over time (reflexive), while a bank uses a structured coding system for reporting (codebook). |
Which One is the Right Approach for Your Business?
Understanding these approaches helps businesses select the most relevant method to generate actionable insights. The right choice depends on several factors:
- Are you exploring unknown issues or validating existing concerns?
Inductive analysis is better for discovering unexpected trends, while deductive analysis works for confirming hypotheses. - Does your research require direct feedback interpretation or deeper meaning extraction?
Semantic analysis is ideal for surface-level trends, while latent analysis helps uncover underlying motivations behind customer responses. - Do you need flexibility or a structured framework?
Reflexive analysis allows for adaptive theme development, whereas codebook analysis ensures consistency and comparability over time.
By aligning the method with business objectives, organizations can ensure their thematic analysis delivers insights that drive meaningful improvements.
AI-Powered Thematic Analysis: The Future of Experience Data Insights
Businesses handle an overwhelming volume of customer insights daily, making it difficult to track patterns manually. AI-powered thematic analysis simplifies this process, enabling faster, smarter, and more effective insights.
Here’s why AI is crucial to make thematic analysis faster, smarter, and more effective:
- Processes large datasets instantly: AI scans customer comments, reviews, and support tickets in seconds, uncovering trends that would take human teams days.
- Eliminates human bias: AI identifies themes directly from data, ensuring objectivity without personal interpretation.
- Detects trends early: AI spots recurring issues before they escalate, enabling businesses to take proactive action.
- Reduces manual effort: Teams can focus on solving problems rather than sorting through data.
- Understands sentiment: AI detects customer emotions in feedback, categorizing responses as positive, neutral, or negative to help businesses prioritize concerns.
With AI-driven thematic analysis, businesses move beyond traditional manual methods, gaining real-time, data-driven insights that improve customer experience and drive business growth.
How to Perform Thematic Analysis with AI Tools Like Zonka Feedback
AI-powered tools streamline thematic analysis by automating data collection, pattern recognition, and insight generation. With Zonka Feedback, businesses can efficiently analyze customer experience data and take action to improve satisfaction.
Here’s how it works:
1. Bring All Your Data Together
Import feedback from multiple sources—surveys, support tickets, chat logs, call transcripts, and user behavior data—to get a comprehensive view of customer experiences.
2. Define Your Focus Areas
Set analysis goals by asking key questions such as "What frustrates customers the most?" or "What product improvements do users expect?" This step ensures that AI uncovers insights aligned with business priorities.
3. AI Detects Key Themes
AI scans through large datasets, identifying recurring themes and subthemes without manual intervention.
For example, if customers frequently complain about slow responses, unhelpful agents, and unresolved issues, AI categorizes these concerns under Customer Support Issues, with subthemes such as:
- Waiting too long for a response
- The agent did not resolve my issue
- No follow-up after a complaint
AI continuously improves its accuracy over time, refining categorizations as it processes more feedback.
4. Refine and Customize Themes
Businesses can merge similar themes, adjust AI-generated categories, or introduce new ones to ensure the analysis aligns with their needs. AI can also flag high-priority concerns, such as product failures or legal issues, enabling immediate action.
5. Get Real-Time Insights
Interactive dashboards and reports help businesses monitor trends, track recurring issues, and uncover pain points before they escalate.
6. Turn Insights into Action
AI-driven insights allow businesses to automate responses for common issues, optimize customer service processes, and improve products. By prioritizing critical concerns, teams can enhance service efficiency, reduce complaints, and create a seamless customer experience.
With AI-powered thematic analysis, businesses no longer need to manually sift through massive datasets. Instead, they can focus on what matters most—understanding customers and making meaningful improvements.
Manual vs. AI-Powered Thematic Analysis
Thematic analysis can be performed manually or with AI, each offering distinct advantages. While manual methods allow for deeper interpretation, AI enables faster and more scalable analysis. Here is a comparison of how both approaches differ across key factors:
Factor | Manual Thematic Analysis | AI-Powered Thematic Analysis |
Understanding & Context | Captures nuanced meaning as humans recognize subtle patterns. | Identifies patterns quickly but may need human oversight for deeper context. |
Flexibility | Themes evolve naturally based on new data. | Users can refine, merge, or create categories for accuracy. |
Speed & Scalability | Time-intensive and impractical for large datasets. | Processes thousands of customer comments in seconds. |
Bias & Accuracy | Prone to subjective interpretation. | Categorizes themes based on statistical patterns, reducing bias. |
Trend Identification | Takes longer to recognize emerging patterns. | Detects trends early, enabling proactive responses. |
Automation & Efficiency | Requires manual effort for coding and categorization. | Automatically generates themes and subthemes. |
Reporting & Insights | Compiling reports is time-consuming. | Provides real-time tracking of trends and concerns through automated reporting. |
While manual analysis is useful for nuanced understanding, AI-powered tools enhance efficiency, accuracy, and scalability, enabling businesses to act on AI customer insights faster.
Thematic Analysis Using Generative AI and NLP
Generative AI and Natural Language Processing (NLP) enhance every stage of thematic analysis by automating categorization and improving accuracy. AI continuously refines its analysis over time, ensuring businesses gain deeper and more relevant insights.
Here’s how they elevate analysis:
→ Contextual Understanding
Unlike traditional keyword-based methods, NLP understands context, tone, and sentiment, ensuring accurate categorization even in complex feedback. AI can distinguish between similar phrases with different meanings:
- "The refund process is easy." → (Positive Sentiment)
- "The refund process should be easy, but it’s not." → (Negative Sentiment)
- "Customer support was helpful." → (Positive)
- "Customer support tried to help but failed." → (Appreciated the effort but still Negative)
This deeper language understanding ensures that themes are categorized accurately, even in complex feedback. Also, AI-powered NLP supports multilingual analysis, accurately capturing sentiment across different languages and cultural expressions.
→ Subtheme and Topic Extraction
NLP models segment broad themes into subthemes and topics, enabling product teams to prioritize improvements based on user concerns. For example, under the theme “Product Usability,” AI may identify subthemes like:
- Interface Issues (unclear navigation, complicated settings)
- Performance Problems (app crashing, slow response time)
- Missing Features (users requesting specific functionalities)
As new trends emerge, AI adapts its categorization, ensuring businesses stay ahead of shifting user concerns.
→ Sentiment and Emotion Analysis
AI categorizes sentiment as positive, negative, or neutral and detects emotions like frustration, satisfaction, urgency, or disappointment. It also recognizes sarcasm and mixed sentiment, distinguishing when feedback contains both positive and negative elements.
💡 For example, a customer leaves a comment: “I’m frustrated with the slow delivery.”
AI classifies it as:
- Theme: Shipping Delays
- Subtheme: Late Deliveries
- Topics: Delivery Speed, Customer Experience
- Intent: Complaint
- Urgency: Medium (affects satisfaction but may not be critical)
- Sentiment: Negative
- Emotion: Frustration
This capability helps businesses prioritize issues based on urgency and customer impact.
→ Scalability and Consistency
AI processes millions of data points in minutes while maintaining accuracy, making large-scale analysis seamless. Unlike manual analysis, which can be inconsistent and time-consuming, AI:
- Maintains consistency by applying the same analysis rules across all data sources.
- Eliminates human bias, ensuring data-driven categorization.
- Integrates with business tools, such as CRM systems and analytics platforms, providing real-time insights for customer service and decision-making.
By leveraging AI-powered thematic analysis, businesses can efficiently track trends, uncover emerging concerns, and enhance decision-making with precise, data-driven insights.
How Thematic and Sentiment Analysis Work Together for Better Insights?
Thematic analysis identifies key topics, but without sentiment analysis, it lacks emotional context—making it unclear whether the feedback is positive, negative, or neutral. By combining both, businesses gain a more holistic view of customer data, allowing for better prioritization and decision-making.
For example, if “Pricing” is a recurring theme in user comments, thematic analysis flags it. But without sentiment analysis, businesses won’t know whether customers see pricing as fair or overpriced. Similarly, if “Customer Service” is trending, sentiment analysis reveals whether it’s seen as exceptional or frustrating.
Factor | Without Sentiment Analysis (Limited Understanding) | With Sentiment Analysis (Complete Insights) |
Context | Identifies key topics but lacks emotional context. | Detects emotion, urgency, and intent, revealing the full picture. |
Efficiency | Requires manual effort to determine whether feedback is positive or negative. | AI automatically categorizes sentiment, making analysis faster and more unbiased. |
Prioritization | Highlights common themes but doesn’t indicate urgency. | Identifies which themes matter most based on customer emotions and urgency. |
Proactive Action | Issues can go unnoticed until they escalate. | Sentiment analysis detects negative trends in real-time, enabling teams to prevent issues from escalating. |
When both methods are combined, businesses don’t just see what’s trending—they see why it matters. This deeper insight helps prioritize the right actions, respond faster, and make meaningful improvement
Best Practices for Thematic and Sentiment Analysis
To turn feedback into meaningful action, businesses need a structured approach. Here’s how to maximize the effectiveness of thematic and sentiment analysis:
- Align insights with business goals: Ensure themes directly tie to objectives like improving retention, reducing customer churn, or enhancing product usability. Pair qualitative themes with quantitative metrics like NPS or CSAT to provide context and justify strategic decisions.
- Use multi-level thematic analysis: Dive deeper into user data by organizing themes and subthemes. For example, under "Customer Support Issues," subthemes like "unresolved tickets" help pinpoint specific problems.
- Combine sentiment and emotion analysis: Understand not just what customers say but how they feel. Identifying emotions like frustration or satisfaction enables proactive problem-solving.
- Track themes over time: Validate the impact of actions, predict customer needs, and benchmark against industry standards to stay ahead of shifting expectations.
- Refine themes continuously: Regularly review and update themes to reflect evolving customer needs. Validate findings with frontline teams for accuracy.
- Foster cross-functional collaboration: Involve CX, product, support, and marketing teams to ensure insights drive actionable changes across the organization.
- Leverage AI insight tools: AI continuously improves by learning from new feedback, refining accuracy, and adapting to evolving language patterns. Advanced AI insight tools provide predictive analytics and hyper-personalized customer analysis.
- Invest in automation: Integrate AI-driven thematic analysis with CRM and support systems to generate real-time insights, enabling teams to act independently.
- Measure impact: Track how addressing specific themes, such as "app crashes" or "billing errors," improves customer satisfaction and retention.
- Scale with AI feedback loops: Integrate AI-driven thematic analysis with CRM and support systems to create AI feedback loops, where real-time insights trigger automated actions, enabling teams to act independently.
- Build a feedback-driven culture: Train employees to act on thematic insights and share findings with leadership to align business decisions.
By following these best practices, businesses can drive customer satisfaction, loyalty, and long-term growth.
The Future of Thematic Analysis with AI
AI-powered thematic analysis is evolving beyond identifying past trends—it is becoming predictive, automated, and deeply integrated into business ecosystems.
- Hyper-personalized insights
AI will tailor feedback analysis to individual users, offering customized insights that enhance engagement and retention. Businesses will gain a granular understanding of customer preferences, allowing them to deliver experiences that feel uniquely personalized.
As AI advances in CX, 70% of leaders believe chatbots are becoming skilled architects of highly personalized customer journeys, highlighting AI’s growing role in delivering tailored customer interactions and insights.
- Predictive analytics and automation
AI will forecast customer issues before they escalate and trigger real-time actions to resolve them proactively. Instead of reacting to complaints, businesses can anticipate pain points and implement preventive measures.
With 70% of organizations actively investing in technologies that capture and analyze intent signals, businesses are increasingly relying on AI to predict customer needs and enhance decision-making before issues arise.
- AI-driven enterprise integration
Future AI-driven thematic analysis will seamlessly integrate with CRM, customer service platforms, and automation tools, ensuring businesses can act on insights instantly. This integration will create an AI feedback loop, where insights lead directly to optimized workflows and customer experiences.
However, as AI becomes central to business strategies, 74% of CX leaders emphasize that transparency is critical, as customers and regulators increasingly demand insight into automated decision-making.
These advancements will redefine thematic analysis, making it faster, more accurate, and highly actionable. Businesses will no longer just analyze customer data—they will predict, personalize, and automate solutions in real time, ensuring a proactive and seamless customer experience.
Conclusion: Turning Customer Data into Action
Throughout this guide, we’ve explored the fundamentals of thematic analysis, from structuring qualitative data and identifying key themes to leveraging AI-powered tools for deeper insights. But the real takeaway is that thematic analysis isn’t just about categorizing feedback—it’s about creating impact.
Businesses that succeed don’t just collect data; they act on it. By continuously tracking trends and addressing customer needs, they enhance experiences, optimize service quality, and build long-term loyalty. AI-powered thematic analysis transforms unorganized responses into clear, actionable insights, enabling businesses to streamline data processing, automate analysis, and make informed decisions.
At its core, thematic analysis is only as powerful as the actions it drives. It’s not just about analyzing feedback—it’s about using insights to create meaningful improvements. By listening to customer voices, taking action on feedback, and refining strategies over time, businesses can turn thematic analysis into a powerful growth engine.
FAQs on AI Thematic Analysis
1. Can Thematic Analysis be used for quantitative data like NPS & CSAT?
Thematic analysis enhances NPS and CSAT by providing qualitative context, helping businesses uncover the reasons behind customer ratings and implement targeted improvements.
For example,
- Without Thematic Analysis: A CSAT score of 3.2/5 tells you customers are dissatisfied but doesn’t explain why.
- With Thematic Analysis: Open-ended responses reveal frustration with "long wait times" and "billing errors," allowing businesses to take action.
Businesses gain deeper insights and actionable strategies to improve customer retention by analyzing text responses alongside survey scores.
2. When should a business consider using Thematic Analysis?
Thematic analysis is essential when businesses need to uncover the root causes behind survey scores and track trends over time to prevent recurring issues. Companies should consider it when:
- They receive large volumes of customer feedback (reviews, support tickets, surveys, chat logs).
- They need to analyze multiple data sources for a holistic customer experience view.
- NPS, CSAT, or CES indicate a problem, but the root cause is unclear.
- They want to predict and prevent recurring issues rather than reactively addressing them.
3. How does AI improve Thematic Analysis for businesses?
AI-powered tools analyze thousands of responses in minutes by automatically detecting themes, subthemes, and sentiment. AI also:
- Processes real-time data for immediate insights.
- Helps detect emerging trends before they escalate.
- Speeds up analysis by categorizing themes automatically.
- Continuously refine accuracy by learning from new feedback and adapting to evolving customer language patterns.
4. Should businesses replace manual Thematic Analysis with AI?
Not entirely. AI enhances efficiency by automating theme identification and sentiment tracking, while human oversight ensures accuracy and contextual refinement. A hybrid approach is the most effective, where:
- AI categorizes large datasets quickly, reducing manual workload.
- Humans validate insights, refine themes, and interpret nuances like sarcasm, industry-specific language, or cultural variations.
This combination delivers the most accurate and meaningful insights, ensuring businesses make data-driven decisions without losing human expertise.
5. Can Thematic Analysis be applied to audio or video data?
Yes! Speech-to-text transcription allows thematic analysis to process spoken feedback. Businesses can analyze:
- Call center recordings to identify recurring complaints.
- Customer interviews to detect common feedback themes.
- Employee feedback from town halls or virtual meetings.
AI-powered voice analytics tools can also classify tone, emotions, and speaker intent for deeper insights.
6. How does Thematic Analysis impact customer loyalty and retention?
Businesses that analyze and address recurring customer concerns create better experiences, leading to higher customer retention. Customers stay with companies that listen and act on feedback.
- For example, a telecom company identified billing confusion through thematic analysis and simplified invoices. This improved communication, increased satisfaction and boosted retention by 5%.
AI-powered analysis helps detect emerging issues early, enabling proactive improvements that reduce churn and enhance loyalty.
7. What are the challenges of Thematic Analysis?
The following are the common challenges:
- Too many themes make prioritization difficult.
- Manual coding is subjective, causing inconsistencies.
- Scaling manually is slow and inefficient.
AI streamlines analysis by categorizing feedback at scale, reducing bias, and refining themes for accuracy.
8. How long does Thematic Analysis take?
Manual analysis can take weeks, requiring extensive reading and categorization, while AI-powered analysis processes thousands of responses in minutes. Most businesses adopt a hybrid approach, where AI detects themes, and humans refine insights for accuracy. With AI-driven real-time insights, businesses can act on trends instantly, enabling faster and more informed decision-making.
9. What tools can be used for Thematic Analysis?
AI platforms integrate with CRM and customer service tools to automate analysis and speed up decision-making. The choice of tools depends on business needs and data volume.
- Manual tools like Excel, Google Sheets, NVivo, and ATLAS.ti are useful for analyzing small datasets and manually categorizing themes.
- AI survey tools such as Zonka Feedback, Thematic, Qualtrics, and Medallia automate theme detection and sentiment analysis, making large-scale feedback processing more efficient.
- NLP and text analytics tools like Python (NLTK, SpaCy), IBM Watson, and AWS Comprehend process large text datasets, enabling deeper language analysis and pattern recognition.
AI tools save time, reduce bias, and provide structured insights for better decision-making.
10. How should businesses present Thematic Analysis results?
AI-powered reports provide real-time insights and recommendations. To present results effectively:
- Use visuals like heat maps and bar charts to highlight trends.
- Link themes to key metrics like NPS and CSAT for context.
- Give clear recommendations to improve operations, support, or products.
Simple, data-backed reporting ensures informed decisions and quick action.